Stripe’s latest move is a bold bet on artificial intelligence. This week, the fintech giant unveiled what it calls the world’s first AI foundation model for payments, a gargantuan machine-learning model trained on its vast trove of transaction data. The company is touting this system as a breakthrough that will make online commerce safer and more efficient – flagging fraud that others miss, while boosting legitimate sales. It’s a headline-grabbing announcement, positioning Stripe at the forefront of AI innovation in finance. But beyond the splashy claims lies a deeper story about how AI is reshaping fraud detection in fintech, the competitive pressures driving such projects, and the thorny regulatory questions they raise on both sides of the Atlantic. In this analysis, we examine Stripe’s new AI model not just as a tech upgrade, but as a sign of the times: a case study in the promise and perils of deploying artificial intelligence at massive scale in the financial system.
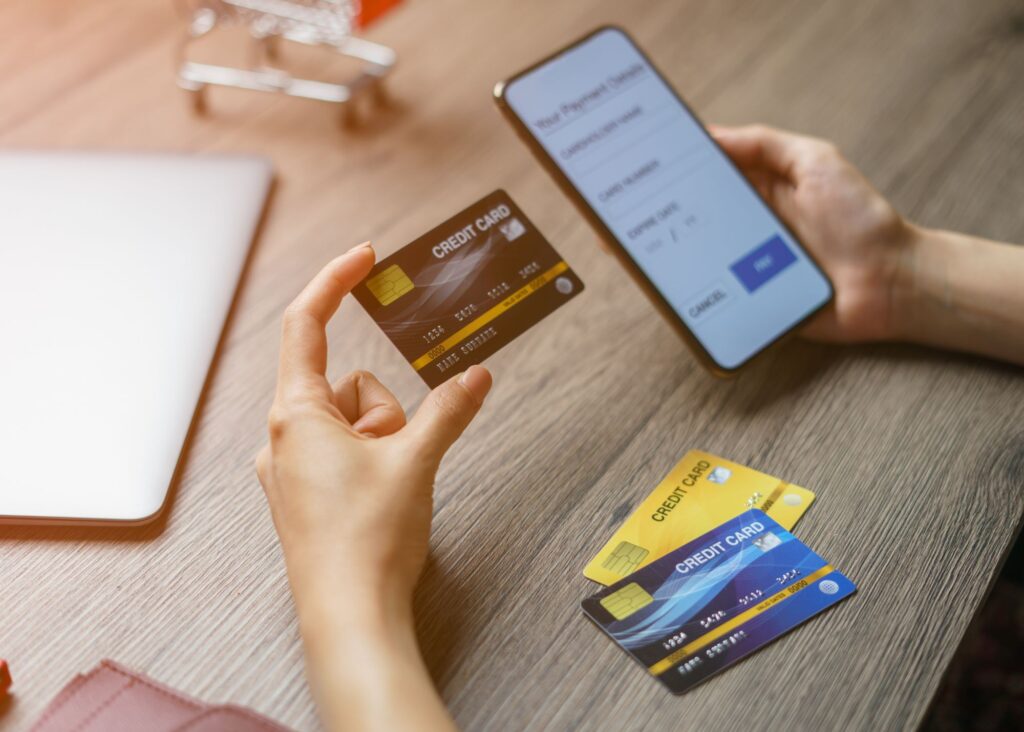
Table of Contents
ToggleStripe’s Foundation Model: AI Meets Payments Data
At its annual Sessions conference, Stripe co-founder and CEO Patrick Collison invoked two “gale-force tailwinds” transforming the economy: AI and stablecoins. Stripe’s strategy, he said, is to harness those forces for its users “right away.” On the AI front, the company’s star launch is the new Payments Foundation Model, an in-house AI system built on an unprecedented dataset of tens of billions of payment transactions. By training on years of global payments flowing through Stripe’s platform (some $1.4 trillion in volume processed in 2024 alone), the model has learned to recognize “hundreds of subtle signals” in each payment that smaller, specialized models might overlook. In other words, this is a generalized AI brain for payments – one that Stripe claims can draw on the full richness of its network’s data to make smarter decisions about which transactions to approve, trust, or block.
How was this model trained? Stripe’s team turned to self-supervised learning, a cutting-edge approach that lets AI discover patterns without strict human labeling. Will Gaybrick, Stripe’s president of product, explained that the model “discovers its own features” from the raw data. Instead of being explicitly told what a fraudulent transaction looks like, the system has essentially taught itself by analyzing billions of examples. The bet is that this broad, foundation-style training will make the AI more adaptable and powerful. “We have found over and over… generalized models outperform,” Gaybrick noted, emphasizing that such models are more agile in adapting to new fraud patterns. Indeed, Stripe’s model is not narrowly tuned to one task – it’s designed to underlie many aspects of payments, from fraud detection to optimizing authorization rates and even personalizing checkouts. Stripe has long used separate AI models for specific purposes (preventing fraud, raising approval rates, recommending the best payment method at checkout). Now, it’s attempting to unify these learnings in one foundation model that will be “deployed across Stripe’s payments suite” to unlock improvements previously out of reach.
What can this AI actually do? Stripe’s first touted use-case is fraud, especially a menace known as card testing. In card-testing attacks, fraudsters rapidly try out stolen card numbers with small purchases to find which cards are valid. Stripe says its prior generation of fraud models already curbed such attacks by 80% over two years, but the new foundation model took things even further. When Stripe recently turned the model loose on transactions from large businesses, it increased detection of card-testing fraud by 64% practically overnight. In fintech, improvements of that magnitude are eye-popping. Emily Glassberg Sands, Stripe’s head of information, summed up the leap: “Previously, we couldn’t take advantage of our vast data. Now we can.” The implication is that older systems, however effective, were siloed or limited – whereas this new AI can finally exploit the full scale of Stripe’s network effect. By capturing nuanced patterns across millions of businesses and billions of payments, it may catch schemes that went undetected before.
Beyond fraud, Stripe hints at other gains. An obvious target is the plague of false declines – legitimate purchases that get wrongly rejected by fear of fraud. Every blocked good transaction means lost revenue and frustrated customers. With its richer analysis, Stripe’s model might approve more good orders that legacy rules or simpler models would have declined. (The company has cited a 2.2% average lift in authorization rates from related AI optimizations in the past, and the foundation model could amplify that.) Other applications include dynamic risk-based authentication – deciding when to ask a customer for extra verification – and even tailoring the checkout experience (for instance, showing the payment methods most likely to succeed for a given customer). All these decisions involve subtle signals (device fingerprints, behavioral patterns, spending history) that an AI of this scale can analyze in real time. Stripe hasn’t released detailed metrics on these fronts yet, but the aim is clear: squeeze more revenue out of the system by fighting fraud and boosting conversion simultaneously. It’s a delicate balance, and Stripe is effectively saying, “Our AI is now smart enough to give you both – more security and more sales.”
The “foundation model” terminology itself is telling. In AI circles, foundation models refer to large-scale models (like OpenAI’s GPT-4 or Google’s large language models) that serve as general platforms for many tasks. Stripe co-opting this term suggests it views its payments AI not as a narrow tool, but as a transformative base layer for its products. It also implicitly claims a first-mover status: no other payment processor has publicly announced an AI model of this scope. Stripe calls it “the industry’s first Payments Foundation Model” – a boastful but not unbelievable claim. While banks and networks have used AI for years (often very successfully), those systems typically focus on specific algorithms for fraud scoring or credit risk. Stripe is signaling that it has something broader: an AI engine that understands payments in a holistic way, perhaps analogous to how a language model understands language. Whether that analogy holds true will be seen in practice, but it certainly marks a new chapter in how fintech approaches machine learning.
AI in Fraud Detection: Fintech’s Ongoing Arms Race
Stripe’s innovation does not emerge in a vacuum – it’s the latest salvo in a long-running arms race between fraudsters and the financial industry, one that has only intensified with advances in AI. In the digital era, fraud prevention has been often described as a game of cat-and-mouse, with each side upping the technological ante. Early online payments fraud in the 2000s was tackled with relatively simple rules and machine-learning models. But as commerce moved largely online and criminals grew more organized, the sheer volume and sophistication of fraud attempts exploded. Today, roughly 1 in 20 online transactions is fraudulent or suspected to be – TransUnion reported that 5.2% of all digital transactions in the first half of 2024 were flagged as potential fraud attempt. Most of those never hit consumers’ radar because the system’s defenses block them. Yet the constant barrages are staggering in scale.
Artificial intelligence has supercharged both sides of this fight. On the offensive side, fraud rings leverage AI to probe and exploit vulnerabilities. A recent Forbes analysis noted how bad actors use bots and AI tools to launch mass attacks, generate realistic fake identities, and even create deepfake voices or documents to trick systems. Last holiday season illustrated this vividly: during Cyber Monday 2024, Visa saw an 85% year-over-year increase in fraud attempts, and a stunning 200% spike during the opening weekend of the shopping season. Why such a jump? Banks and analysts attribute it in large part to criminals deploying AI – automating and scaling up their scams at unprecedented levels. It’s a dark mirror of what’s happening in many industries: AI empowering malicious behavior just as it empowers legitimate business.
The defenders, in turn, have been forced to raise their game with AI as well. Traditional anti-fraud measures – think static rules like “flag transactions over $X from country Y” – are far too brittle and slow against AI-augmented attackers. Thus, banks, payment networks, and fintechs have invested heavily in machine learning that can adapt and spot anomalies. Visa, for example, was an early pioneer in using neural networks to detect credit card fraud at the point of sale, and it continues to lean on AI for its fraud prevention services. The company said it helped block $40 billion worth of fraudulent transactions from October 2022 to September 2023 through its AI-driven systems. At Visa’s high-security Fusion Center, teams monitor transactions worldwide with the help of 115 different cybersecurity and fraud detection tools, many infused with AI. They’ve built behavioral analytics platforms and “fusion” algorithms that combine signals from hundreds of sources to stop suspicious patterns in real time. Mastercard, not to be outdone, just last year spent $2.65 billion to acquire an AI-centric cybersecurity firm, Recorded Future, to bolster its fraud and cyber intelligence capabilities. (Mastercard had already partnered with that firm to double the detection of compromised card numbers, illustrating how critical AI has become to staying ahead of breaches.)
Fintech startups are also swarming into the fraud prevention space, bringing specialized AI solutions. A glance at Forbes’ 2025 Fintech 50 list shows several anti-fraud upstarts: Alloy, DataVisor, Persona, SentiLink, Zip – all focusing on different niches of financial fraud. DataVisor, for instance, uses unsupervised machine learning to find correlations in seemingly unrelated events that might indicate new fraud rings. This is quite similar in spirit to what Stripe’s foundation model aims to do – detect hidden patterns without pre-labeled examples. SentiLink uses AI to combat identity fraud (e.g. fake or synthetic identities used to open bogus accounts). Sardine, another startup, brands itself as an “AI risk platform” for fraud and compliance and recently raised $70 million, highlighting investor confidence that AI can crack these tough problems.
In short, AI has become the weapon of choice in payment fraud management, and the competition is fierce. Stripe’s move to build a foundation model is the logical next escalation. Previously, Stripe (like others) relied on multiple bespoke models – one to evaluate transaction risk (its Radar service), another to optimize how transactions are routed or retried for approval (its Adaptive Acceptance feature), etc. Those tools already gave Stripe’s merchants an edge; for example, Stripe’s adaptive authorization tech reportedly lifted conversion by about 1% for a major user with no extra effort. But with fraud losses and attack volumes relentlessly rising – global e-commerce fraud losses hit $44 billion in 2024 and are projected to more than double to $107 billion by 2029 – incremental gains are not enough. The “constant arms race” cited by Juniper Research’s analysts requires bigger guns. A foundation model that continuously learns from over a trillion dollars in annual payment activity might be Stripe’s big gun.
Crucially, Stripe’s advantage is its data scale and diversity. While a bank sees only its own customers’ transactions, and Visa sees many transactions but primarily card-based and with limited metadata, Stripe sits at a nexus of millions of businesses, various payment methods, and global consumer behavior. It processes everything from a $5 rideshare fee in London to a $50,000 SaaS invoice in California, across cards, bank debits, digital wallets, and now even crypto payments. Patterns that might be invisible in a smaller dataset can surface when you aggregate at Stripe’s scale. The company notes that it has seen 92% of all credit cards at least once before on its network – a phenomenal coverage that means Stripe’s model likely recognizes a customer’s card and behavior even if they use it at a new merchant. This network effect is a powerful defense: fraudsters can’t easily use the same stolen card at ten Stripe merchants without the AI catching on by the second or third attempt, because it “remembers” what it has seen across the network.
By deploying the new model across every transaction on Stripe, the feedback loop tightens. Each attempted scam teaches the AI something that can immediately benefit all other merchants. This kind of collective intelligence is what Stripe hopes will set it apart from more siloed approaches. It’s akin to having a global immune system for payments: one merchant’s encounter with a fraudster can immunize thousands of others in advance. Stripe isn’t alone in this philosophy – many payment processors and anti-fraud consortiums share data for this reason – but Stripe’s fully integrated platform (where it controls the payment processing and the fraud detection in one place) gives it an ability to deploy changes fast and at scale.
Yet, this arms race dynamic also underscores a sobering reality: as AI improves fraud prevention, criminals will adapt with their own AI, leading to diminishing returns over time. Today’s 64% overnight improvement might shrink a year from now if fraudsters find new weaknesses or even attempt to poison the AI with deceptive inputs. The history of fraud tech is indeed cat-and-mouse: every major advancement (CVV codes on cards, chip cards, device fingerprinting, machine-learning risk scoring) eventually encounters countermeasures or new fraud vectors. Stripe’s foundation model is a significant leap, but it won’t end the war. It might, however, force the adversary to spend more resources and time for each dollar of fraud – which can tilt the economics in favor of the good guys, at least for a while. In the broader context, it shows how fintech firms now feel compelled not just to use AI, but to push AI to its limits in order to stay ahead of a rapidly evolving threat landscape.
Raising the Stakes in a Competitive Payments Landscape
Stripe’s AI announcement is not just a technological milestone; it’s also a strategic maneuver in an intensely competitive payments industry. The company operates in an arena with giants like Visa and Mastercard on one side, and fast-moving fintech rivals like Block (Square) or Adyen on the other – not to mention big banks and countless startups. In this landscape, superior fraud prevention and payment optimization can be a key differentiator. By loudly declaring its leadership in AI, Stripe is sending a message to both customers and competitors: we have the smartest platform – use us if you want the benefits.
Consider the incumbent card networks, Visa and Mastercard. They are fundamentally partners to Stripe (since Stripe processes Visa and Mastercard transactions), but they’re also offering their own value-add services to merchants and banks that encroach on what Stripe does. Visa, for example, sells risk scoring services to issuing banks and merchants based on its global view of transactions – effectively an AI-driven product to predict fraud. Visa proudly notes it was the first in the payments industry to use AI, leveraging it as far back as the 1990s to detect fraud patterns. As discussed, Visa’s current systems block billions in fraud and boast extremely high accuracy, scanning hundreds of factors in each transaction within milliseconds. Mastercard similarly has its SafetyNet and Decision Intelligence products that use AI to assess fraud risk on transactions before they’re approved. In public forums, Visa executives have framed their role as being an AI-enabled guardian of the payment ecosystem, highlighting how they stopped an 85% uptick in fraud attempts last year using advanced models. In short, the networks are heavily invested in being seen as the leaders in payment security.
Stripe’s move challenges that narrative subtly. If Stripe’s own model can detect fraud better or earlier than the network’s tools, merchants and card issuers might rely more on Stripe’s judgement (and data sharing with networks might become more reciprocal). For instance, if Stripe catches a card-testing attack pattern across multiple merchants, it could alert Visa or automatically decline those authorizations even if the issuer’s system hasn’t flagged them yet. There’s a bit of competitive tension here: Stripe is performing risk assessment on transactions before they even reach the card network or bank in some cases. Historically, many merchants (especially smaller ones) have relied on whatever basic fraud screening their payment gateway or bank provided. Stripe’s promise is a premium level of protection baked in, thanks to its AI. That can make Stripe’s service more attractive than a legacy processor that might let more fraud through or decline more good orders.
Meanwhile, fintech rivals like Block (which owns Square) and Adyen are surely racing down a similar path, even if they haven’t made announcements as splashy. Square’s merchant platform has a product called Risk Manager and undoubtedly uses machine learning to monitor transactions for its millions of small-business users. Adyen, a European payments processor at similar scale to Stripe, launched an AI-powered suite called “Adyen Uplift” that optimizes payment authorization and fraud controls, claiming a 6% conversion boost for merchants. Adyen emphasizes network-wide insights and machine learning in its risk tools as well, sounding quite like Stripe’s pitch. These companies recognize that merchants care deeply about two things: maximizing successful sales and minimizing chargebacks/fraud losses. Deliver on those, and you win business. Neglect them, and clients will jump ship to a competitor who does better.
Stripe’s foundation model is thus a play to raise the bar. By quantifying its gains (e.g. “64% more fraud caught overnight”) and tying them to an innovative AI approach, Stripe is attempting to seize the mantle of the most advanced payments platform. This puts pressure on others: if Block or PayPal or legacy acquirers can’t match Stripe’s fraud loss rates or approval rates, they may start to look inferior. We may soon see similar announcements from others in response – perhaps not claiming a “foundation model,” but touting new AI upgrades of their own. In fact, the ripple effect is already visible: just days after Stripe’s news, another fintech announced AI improvements to its verification tech, and companies like Revolut are rolling out AI-based security features. Competition in fintech has an infectious quality – once one player publicizes a breakthrough, others rush to assure customers they too are innovating.
For legacy banks that process payments or issue cards, Stripe’s move is a wake-up call as well. Banks have traditionally leaned on third-party vendors (like Falcon by FICO or services from the card networks) for fraud detection algorithms. Those systems are effective but can be slow to evolve. Now a tech-forward firm is demonstrating what an in-house, modern AI trained on a colossal dataset can achieve. Banks and older payment processors might not have the internal data science chops or unified data to replicate Stripe’s approach easily. This raises an interesting competitive dynamic: Stripe could potentially begin to offer its fraud-fighting capabilities as a service to others. (For example, its Radar fraud detection is currently available mainly to Stripe users, but one could envision Stripe packaging its AI risk scores for external banks or merchants via APIs in the future, which would encroach on vendors’ territory.) In essence, Stripe is turning its massive data advantage into a machine learning advantage, something large banks technically have the data for, but often lack the platform to harness globally.
It’s also worth noting the partnership angle in Stripe’s announcement. Alongside the AI news, Stripe revealed a deepening relationship with Nvidia, the chipmaker synonymous with AI computing. Part of that was a customer story – Nvidia migrated to Stripe’s billing software in record time – but there’s likely more to it. Training a foundation model on billions of transactions is computationally intensive; it presumably required significant GPU horsepower (the kind Nvidia provides). Stripe’s mention of this partnership hints that it’s investing seriously in AI infrastructure. Aligning with Nvidia could give Stripe early access to cutting-edge AI hardware or optimization techniques, which smaller competitors might struggle to afford or implement. In the AI era, owning or accessing superior compute resources can be as important as owning data.
From a market positioning standpoint, Stripe’s AI and stablecoin initiatives also send a message to investors as it eyes an eventual public listing. Stripe has been one of the world’s most valuable private tech companies, and demonstrating new avenues of growth and technological leadership is key to sustaining that narrative. By diving into AI and crypto (stablecoins) in one swoop, Stripe taps into two of the hottest trends in tech and finance. It signals that even as a 13-year-old company, it can reinvent aspects of itself to stay ahead. Competitors will surely respond, but for now Stripe has grabbed the spotlight, and possibly a perception (deserved or not) of being a step ahead of the pack.
U.S. Regulators Take Stock: Privacy, Explainability, and Bias
Amid the excitement over Stripe’s AI model, there’s a quieter subplot unfolding: regulators and watchdogs will be closely scrutinizing how this powerful new system operates. In the United States, financial regulators have been growing increasingly vocal about the use of AI in sensitive domains, and payments fraud detection ticks many boxes – it involves consumer data, it can significantly affect individuals and businesses, and it operates largely as a black box. While the U.S. doesn’t yet have a comprehensive AI law or a financial-specific AI regulation akin to what Europe is rolling out, agencies like the Consumer Financial Protection Bureau (CFPB), Federal Trade Commission (FTC), and banking regulators have all signaled concerns about data privacy, model explainability, and algorithmic bias in AI-driven financial services.
Data privacy is a foremost issue. Stripe’s model is trained on an enormous swath of personal and transactional data: card numbers, names, IP addresses, device IDs, purchase histories – essentially a mosaic of many individuals’ financial behavior. Consumers whose data contribute to this model likely have no idea their information is being used in this way; they simply interacted with a merchant, and behind the scenes Stripe aggregated their data into its AI training pipeline. Is this allowed? Legally, Stripe covers it in their privacy policies – for instance, Stripe’s own privacy center explicitly states that “personal data is required to train Stripe’s fraud and loss prevention models” and that Stripe uses data from across its platform to improve services like fraud detection. In other words, when you pay via Stripe, your data can be utilized to help train models that might be deployed for fraud prevention (which indeed benefits you and others). U.S. law doesn’t outright forbid this kind of internal data use, especially when it’s under the umbrella of fraud prevention or service improvement that the user implicitly agreed to by using the service.
However, regulators will ask whether Stripe is doing enough to protect and anonymize that data. Stripe says it uses pseudonymized or aggregated data when sharing insights externally, but within the model’s training, raw personal details might be processed. The FTC has been clear that even for AI, companies must adhere to their published privacy commitments and not use data in unexpected ways that could harm consumers. If Stripe’s model were ever to be repurposed beyond fraud (say, for marketing analytics) without proper consent, it would raise red flags. Even sticking strictly to fraud prevention, the sheer scope of data processing could draw scrutiny under laws like the California Consumer Privacy Act (CCPA) or others that give consumers rights over their data. For instance, if a California consumer knew Stripe had their info, they might request it be deleted – how would that square with being part of a trained model? These are new legal frontiers. U.S. regulators might not have clear rules yet, but they are certainly watching. In fact, the FTC in 2020 warned AI developers that training algorithms on biased or improperly obtained data could run afoul of consumer protection laws, and earlier this year it reiterated that companies must address known risks like bias and privacy in their AI models.
Explainability (or rather, the lack thereof) is another big concern. Stripe’s foundation model is undoubtedly complex – likely a deep neural network with millions of parameters. Such models are often described as “black boxes” because even their creators cannot fully explain the rationale behind a given decision. In Stripe’s context, that means if the AI flags a transaction as fraudulent and declines it, neither the merchant nor the cardholder may easily get an explanation beyond a generic “suspected fraud” reason code. Financial regulators worry about this opacity, especially as AI decision-making becomes more autonomous. For example, U.S. banking regulators (the Federal Reserve, OCC, etc.) require banks to have solid model risk management practices – this includes understanding a model’s limits and monitoring its performance. When models are too opaque, banks must take extra steps like parallel runs and human review to ensure things don’t go awry. While Stripe is not a bank, if its AI inadvertently starts denying a lot of legitimate transactions or flags certain customers mistakenly, Stripe could face pushback from its partners (e.g. banks that issue the cards) or even regulators if merchants complain en masse. We’ve seen scenarios where payment platforms using fraud algorithms have terminated or frozen accounts of small businesses without clear explanation, leading to reputational damage and calls for more transparency.
The concept of “model explainability” is gaining traction in regulation. The Securities industry regulator FINRA has noted that some cutting-edge AI applications present explainability challenges, and that an appropriate level of explainability is important especially for autonomous decision-making systems. One can imagine the CFPB – which oversees fair lending and payments – asking Stripe: How do you ensure your fraud model’s decisions are fair and can be explained or justified if scrutinized? Stripe might answer that they validate the model extensively and have human oversight on its rules (for instance, Stripe’s fraud team can analyze feature importances or use tools to interpret the model in aggregate). But in individual cases, the opacity remains. If a consumer wanted to contest a decision (say their legitimate purchase kept getting blocked by Stripe’s system), there is currently no legal requirement akin to credit adverse action notices for fraud screening. Yet, if AI-driven denials started affecting certain groups disproportionately, it’s not hard to foresee regulatory intervention demanding more accountability.
That leads to the third issue: bias and fairness. Could Stripe’s model unintentionally discriminate or create biased outcomes? It’s trained on real-world data, and real-world fraud patterns can correlate with demographics or geography in uncomfortable ways. For example, if historically more fraud has come from certain regions or card types, the model might grow overly suspicious of transactions with those traits – even if they are perfectly legitimate. Without careful checks, automated systems can reinforce biases present in training data. Financial regulators in the U.S., like the CFPB and even state attorneys general, are attuned to algorithmic bias because it can violate anti-discrimination laws (for instance, if an algorithm unfairly disadvantages people from a certain zip code, it could raise Equal Credit Opportunity Act issues by proxy, even if fraud prevention isn’t credit, it’s adjacent to access to services). The FCA in the UK and other global regulators have explicitly researched AI bias and encouraged industry to develop ways to detect and mitigate it. In the U.S., agencies have hinted they won’t hesitate to use existing laws to punish biased AI outcomes.
Stripe, for its part, has not publicly detailed how it tackles bias in its model. One would hope they are testing the model for disparate impact – e.g., ensuring that transactions for certain ethnic-sounding names or from predominantly minority neighborhoods aren’t being falsely declined at higher rates than others without a fraud basis. The challenge with fraud models is that fraud itself is not evenly distributed: international transactions do carry higher risk on average; certain payment methods do get abused more by criminals. Drawing the line between legitimate risk-based differentiation and unfair bias is tricky. Regulators might press for “explainable AI” or at least auditable AI, where Stripe could demonstrate how decisions are made and that they’re grounded in objective risk factors rather than proxies for protected characteristics. This is easier said than done. As FINRA’s guidance suggests, firms may need to isolate variables in the model to see their impact on outcomes and guard against models picking up spurious correlations. For instance, if the model learned that transactions at certain postal codes are risky (perhaps because historically more fraud happened with shipping addresses in those areas), that could inadvertently harm honest consumers living there – a form of “location bias.” Stripe may need to constantly evaluate and tweak the model to ensure it’s accuracy and fairness.
Finally, a point on regulatory oversight: Payment processing and fraud prevention largely fall under the purview of financial regulations (like anti-fraud guidelines, card network rules, etc.), but AI models introduce “model risk” which regulators may view through the lens of safety and soundness. The Office of the Comptroller of the Currency (OCC) has model risk management guidance that banks must follow; while Stripe as a tech firm isn’t directly bound by it, if something goes wrong (say a wave of false declines costing businesses money, or a systemic failure of the model), the impacts could draw in regulators indirectly. Additionally, if Stripe’s model is used in contexts that touch lending or credit decisions (perhaps not now, but conceivably in extending financing or screening users), then fair lending laws would formally apply.
At present, the U.S. relies on a patchwork of laws – data privacy mostly at state level, anti-discrimination, and general consumer protection – to keep AI in check. We can expect U.S. regulators to scrutinize companies like Stripe through enforcement rather than broad rules (at least until any AI-specific regulation is passed). That means Stripe must self-regulate prudently: handling customer data with care, monitoring its model for biases and errors, and being responsive if concerns are raised. The CFPB’s director has warned fintechs about “black box algorithms” leading to illegal discrimination, and the FTC has a keen eye on AI claims and misuse. Stripe likely knows this and will aim to stay in regulators’ good graces by emphasizing the consumer benefits (fraud prevention) while quietly ensuring they have guardrails internally. In summary, in the U.S., Stripe’s AI may not face a specific AI law, but it will operate under the watchful eye of existing regulatory principles – privacy, fairness, transparency, and accountability – all of which are being reinterpreted for the AI age.
Different Rules Across the Pond: Europe and UK’s AI Frameworks
While the U.S. navigates AI through existing laws, Europe is moving toward a more prescriptive regulatory regime for artificial intelligence – something Stripe and peers will have to heed, given their global footprint. The EU’s upcoming AI Act is set to be the world’s first comprehensive AI law, and it takes a strict risk-based approach. Systems used in fraud prevention and financial services are likely to be classified as “high-risk AI systems” under this Act. If Stripe’s payments AI is deployed in Europe, it could fall in that category, meaning Stripe would have to meet a slate of requirements: rigorous risk assessments, documentation of the model’s design and purpose, transparency to users, human oversight measures, and even conformity assessments before deployment. In practical terms, Stripe might need to keep technical documentation explaining how the model was trained, what data was used, and how it mitigates foreseeable risks. European regulators may require that the system be auditable – perhaps necessitating Stripe to provide summary explanations or factors contributing to a fraud decision, at least to business users if not end customers.
One key aspect of the EU AI Act is addressing bias and fairness as a legal compliance matter. If an AI system in payments was found to systematically disadvantage a certain group of people in Europe, that could be deemed non-compliant with the Act’s requirements for high-risk AI, which include ensuring “the absence of bias” in training data and outputs where possible. Stripe would thus need to demonstrate that it has procedures to test for and correct bias in its model when operating in the EU. Additionally, GDPR (the EU’s data protection law) already imposes constraints: it gives individuals the right not to be subject to a purely automated decision that significantly affects them, including decisions related to fraud screening. There is an exemption for fraud prevention (“necessary for the performance of a contract” or the controller’s legitimate interests, perhaps), but even so, GDPR would require that Stripe only uses the minimum necessary personal data and that it protects that data carefully. European regulators (and consumers) might also ask for more transparency into how their data is used. In theory, a European cardholder declined due to Stripe’s model could inquire or complain to a data protection authority, triggering an investigation into the algorithm’s functioning under GDPR’s provisions about automated decision-making and profiling.
Moreover, Europe’s emphasis on explainability and user rights means Stripe might need to adapt how it communicates with merchants and maybe indirectly consumers about its AI. The EU AI Act will likely mandate some level of notice that AI is being used in high-risk situations. So European consumers could gain more visibility or recourse if the AI flags them. All of this suggests a heavier compliance load: Stripe may need to have an “EU mode” for its AI, with more human oversight or the ability to pull an override if the model makes a questionable call. It’s not far-fetched to imagine European regulators insisting on a human appeal process for someone who feels wrongly blocked by an AI-driven system, for example.
Across the channel, the UK is charting its own path on AI regulation, one that so far is more principles-based and flexible. The UK government has signaled a “pro-innovation approach” – avoiding a single omnibus law like the EU’s, and instead empowering sectoral regulators (like the Financial Conduct Authority, FCA, for finance) to apply existing principles to AI. The Bank of England and FCA, however, have not been idle. They have conducted surveys on AI use in finance and issued discussion papers on the potential risks. Notably, the Bank of England has flagged model complexity, lack of explainability, and data bias as fast-growing risks in financial services, directly acknowledging that as firms adopt machine learning, they must guard against these pitfalls. The UK regulators tend to encourage best practices rather than enforce hard rules upfront. For instance, the FCA’s research into AI bias (as seen in its published notes) is meant to guide firms on where to be cautious. The FCA has also introduced the idea of a “Consumer Duty” – requiring financial firms to ensure good outcomes for customers. One could argue that if Stripe’s model (as a part of a payment service) systematically caused poor outcomes for certain customers (like unnecessary declines or delays), a firm operating under UK jurisdiction might be expected to adjust it under those consumer protection principles.
So how might Stripe’s approach differ in the UK/EU versus the U.S.? For one, documentation and governance will be key in Europe. Stripe will likely have to maintain detailed documentation of its model’s development and testing to satisfy EU requirements. It might also have to allow European clients some configuration or at least information – for example, maybe giving merchants more insight into why a transaction was flagged (so the merchant can relay or check, which aligns with transparency goals). The UK might push for explainability in a softer way: regulators there could ask in supervisory conversations, “How do you ensure your model’s decisions can be explained to an impacted customer if needed?” Even absent hard law, not being able to answer that could be a competitive disadvantage as banks and merchants might prefer AI tools that they can interpret or justify.
Another aspect is data locality and usage constraints. European laws might restrict how Stripe can transfer European personal data for model training. If Stripe trained this model using global data (including EU transactions), it would need to ensure GDPR compliance in those transfers (using mechanisms like standard contractual clauses, etc.). Stripe does have an Irish entity (Stripe Payments Europe) which acts as a data controller for EU data, and it likely processes EU data in Europe or under strict rules. The regulatory trend in Europe is to possibly demand that high-risk AI involving EU citizens be trained on data that meets EU standards for consent and privacy. Stripe will be mindful of that, perhaps even considering training regional variants of the model if needed to appease regulators (though that would sacrifice some global learning benefit).
Notably, European regulators see fraud prevention as important, but they won’t exempt it from AI rules entirely. In fact, the current draft of the EU AI Act’s high-risk use-cases includes AI for “creditworthiness assessments” and other financial services; fraud detection could easily be interpreted as high-risk too, given its impact on individuals’ access to services. Some EU voices have even suggested that sensitive uses of AI should come with mandatory transparency to users and an option for human review. So, we could envision that Stripe might eventually have to provide EU consumers a channel to contest an automated fraud decision, or at least provide an explanation if asked. This is speculation, but directionally it’s where policy is headed – making AI accountable.
Meanwhile, the UK’s lighter approach could change if the EU’s strict regime proves effective or if a high-profile AI failure occurs. The UK is balancing innovation with safety; it doesn’t want to drive fintechs away with heavy rules, especially since London is a fintech hub. Stripe, being a major player serving UK businesses, would likely engage with UK regulators to demonstrate it’s managing risks voluntarily. The Bank of England’s point about data is quite interesting: it found that four of the five top risks with AI relate to data, and that data privacy is seen as the biggest regulatory barrier to AI adoption in finance. This suggests UK firms (and by extension Stripe) are concerned that unclear rules on data use will hinder them. Ironically, this favors big players: “Without clear rules, larger firms with proprietary data and legal firepower can forge ahead, while smaller players risk being left behind,” the BoE observed. Stripe, of course, is one of those with huge proprietary data and resources to navigate regulation. So in the UK/EU regulatory chessboard, Stripe’s massive data-trained model might actually entrench its advantage – it has the means to comply with complex regulations, whereas a startup with less data might not even attempt such an AI due to compliance overhead.
In sum, Stripe will have to juggle different regulatory expectations: more transparency, documentation, and human oversight in Europe and the UK; more self-policing and broad principles in the U.S. Failure to do so could invite fines or restrictions, especially in the EU. Success in doing so, on the other hand, could make Stripe a trusted leader in AI-driven finance globally. It’s a high-wire act: push innovation, but don’t trip the wires of privacy laws or AI ethics guidelines. The roadmaps regulators are sketching out will heavily influence how far and fast Stripe can roll out its AI model worldwide.
Implications for Startups, Developers, and Stripe’s Users
What does Stripe’s AI leap mean for the thousands of businesses and developers that rely on Stripe – and for the fintech ecosystem at large? In principle, Stripe’s customers stand to benefit significantly from this move. Online businesses big and small care deeply about two metrics in payments: their authorization rate (what percentage of legitimate customer payments succeed) and their fraud loss rate (how much fraud gets through, resulting in chargebacks or losses). Stripe is effectively promising to improve both metrics through its AI. A higher auth rate means more sales completed; lower fraud means fewer chargeback fees and headaches. If Stripe’s foundation model delivers as advertised, a merchant using Stripe could see more revenue with no extra effort – it’s as if the entire Stripe network becomes smarter and that intelligence is a rising tide lifting all boats.
For example, a small e-commerce startup using Stripe might today have to manually review some transactions or set basic rules in Stripe’s Radar (like blocking high-risk countries). With the new AI, they might find the system catches fraud more accurately without overly blocking good orders. Stripe mentioned that in early tests, it caught a wave of card-testing fraud instantly that would have otherwise required months of incremental learning. That kind of immediate response is invaluable to a startup that could be blindsided by a fraud attack. In practice, this means less time and money spent on fraud operations for Stripe users. Many businesses don’t have dedicated risk teams; they rely on Stripe to be that shield. Now that shield is thicker.
Enterprise clients – large marketplaces, SaaS companies, on-demand services – similarly could see improvements. These companies often measure basis-point changes in approval rates in millions of dollars. (Recall that Stripe has publicized that its machine learning tools can increase revenue by around 1% on average via better conversions, which for a company processing $1 billion is $10 million more in sales.) If the foundation model’s holistic approach yields, say, an additional 0.5% boost in genuine transactions approved (by reducing false declines) and a reduction in fraud disputes by a similar margin, that’s material. And it comes without the enterprise having to integrate or pay for a separate fraud solution – it’s built into Stripe. This raises the competitive pressure on standalone fraud prevention vendors: why pay for a third-party fraud tool if Stripe’s default is so good? Many Stripe enterprise users already use its Radar and other tools, but some layered additional vendors for safety. Stripe’s message now is “you might not need those extras; our AI has you covered.”
For developers and startups building on Stripe, another potential implication is new features or products leveraging this AI. While Stripe hasn’t announced a direct API to the foundation model (and likely won’t expose the raw model), we might see new endpoints or data coming out of it. For instance, Stripe could provide merchants with more detailed risk insights: maybe an API that tells a merchant, “This transaction is deemed very high risk and here are the contributing factors,” allowing the merchant’s app to decide how to handle it (e.g. require additional user verification). Developers might also see expanded automation – Stripe’s Radar could introduce more automated actions or suggestions powered by the foundation model’s understanding. Already, Stripe has a feature where it will automatically retry a declined payment at an optimal time or alter how it submits it to the bank (Adaptive Acceptance) – those decisions could become more finely tuned by the new AI. For developers running subscription businesses, this could mean fewer involuntary churns due to payment declines, which is a big win.
However, one should also consider the potential downsides or trade-offs for Stripe users. They are, in effect, ceding a lot of control to Stripe’s black box. While trusting Stripe has generally paid off (few companies want to build their own fraud system from scratch), reliance on one AI model means if it makes a systematic mistake, many businesses could be affected simultaneously. Imagine a scenario – purely hypothetical – where the model due to some learned quirk starts falsely flagging a popular legitimate behavior as fraud. This could lead to many customers unable to make purchases across different merchants until Stripe corrects it. For a developer or business, that’s a dependency risk. In the past, some businesses kept manual review or third-party checks as a fallback. They might still want to, especially during the foundation model’s early days, until trust is earned. Stripe did not specify the model’s false-positive rate or how much it reduces legitimate transactions being blocked. It highlighted catching more fraud, but an unanswered question is whether it does so without casting a wider net that might snag some good users. For most, Stripe’s track record suggests it will improve overall outcomes, but savvy enterprise clients will likely monitor their metrics closely after the model’s full deployment.
For fintech startups in the fraud detection arena, Stripe’s announcement is a double-edged sword. On one hand, it validates the approach of using massive data and AI – which is what many of them do in niche areas (like SentiLink with identities, or Alloy with onboarding). On the other hand, it signals that the infrastructure players like Stripe are embedding advanced AI into their platforms, possibly encroaching on territory that specialized startups target. A merchant who might have considered buying an add-on fraud solution might now simply stick with Stripe’s out-of-the-box offering. This consolidation of capability could make it harder for standalone fraud-tech startups to gain market share, unless they offer something Stripe can’t (or won’t) do – perhaps more customization, or catering to non-Stripe payment flows. It’s a reminder that platforms tend to absorb functions over time (like how Amazon built in-house AI for recommendations rather than relying on a third party). Startups in fintech will need to focus on areas Stripe doesn’t cover or customers it doesn’t serve (for instance, non-Stripe users, or banks) – or perhaps align with Stripe’s ecosystem rather than compete.
Interestingly, Stripe’s move might also open new opportunities for developers and entrepreneurs: with better fraud prevention in place, one could build higher-risk innovative services on Stripe that previously might have been too risky. For example, someone might create a marketplace for a high-fraud category (say tickets or gift cards) and trust Stripe’s AI to handle much of the fraud, whereas before they’d have shied away or spent heavy resources on fraud management. Stripe is basically offering its clients a kind of fraud insurance via AI – not literally insurance, but a safety net that allows them to focus on business growth. This democratizes advanced risk tech: even a two-person startup can now leverage a model trained on billions of transactions. In that sense, Stripe is empowering developers by abstracting a very complex problem (fraud detection) and solving it at platform level.
For end consumers, the impact should be mostly positive but somewhat invisible. Ideally, they experience smoother checkouts – fewer times where a legitimate card is inexplicably declined, fewer calls from their bank asking to verify a purchase that was actually fine. They might also be spared from having their card misused at all, because Stripe caught the fraudster testing it. On the flip side, consumers may notice a bit more intelligent friction: for instance, Stripe’s model might trigger an extra verification step in certain cases (maybe it texts them a code via their bank’s 3D Secure if something looks off). But those steps should target truly risky situations and thus prevent bigger problems. Consumers likely won’t know an AI is watching behind the scenes – if Stripe does its job well, it fades into the background, simply making digital commerce feel more trusted.
Finally, one cannot ignore the implication for innovation and new products on Stripe. With such a powerful model at its core, Stripe could explore entirely new services. Perhaps offering credit or financing with better risk modeling, or dynamic pricing or personalized offers based on customer segments (they did mention improving user experience and identifying services users might need). The foundation model could become a base for more than just fraud: it might inform marketing analytics, or help Stripe advise a business on optimizing payment acceptance. For now, Stripe is framing it around fraud and payments performance, but knowing Stripe’s product cadence, they will leverage this asset widely. Developers on Stripe’s platform might soon get access to more AI-driven analytics – for example, predictive insights (“customers similar to this one tend to prefer payment method X”) or automated routing (“send this transaction via an alternate payment rail because it’s likely to fail on the primary one”). All of which circles back to benefiting those building on Stripe, giving them superpowers without needing in-house data science.
In summary, for Stripe’s users – whether a tiny webstore or a Fortune 500 company – the new AI model promises a safer, more lucrative payments experience largely by outsourcing complexity to Stripe’s intelligence. It levels the playing field, as even the smallest business can harness a model trained on a trillion dollars of data. But it also further ties their fortunes to Stripe’s decisions, which means trust in Stripe must be very high. Given Stripe’s prevalence (it serves millions of businesses and reportedly over 70% of top AI startups), this move could quietly raise expectations across the board: soon merchants might demand their payment providers have “Stripe-like” AI performance, or else consider switching. In that way, Stripe’s innovation could lift standards industry-wide, forcing everyone to step up or plug in to equivalent networks.
Unanswered Questions and Concerns
Stripe’s announcement, as comprehensive as it was, left a number of important questions dangling. It’s common for companies to trumpet the positives of a new AI system, but the real test lies in how they address the nuanced challenges. Here are some key concerns that Stripe did not explicitly answer:
- How does the model balance fraud prevention vs. false positives? Catching 64% more fraud is great, but not if it also erroneously blocks a bunch of legitimate transactions. Stripe hasn’t shared data on whether the new model reduces false declines. Will customers see fewer inexplicable “transaction declined” messages, or could there be instances where the model, being aggressive, turns away good sales? Maintaining a low false-positive rate is as crucial as improving the true-positive rate in fraud detection – merchants care about both sides of that coin.
- What exactly are the “hundreds of subtle signals” the model uses? While Stripe won’t divulge its secret sauce, an outline of the types of signals could reassure users. For example, are these signals purely transactional (amount, merchant category, time of day), or do they include behavioral and device data (typing speed, device gyroscope info, etc.)? Understanding this helps assess whether any signals might inadvertently be proxies for sensitive attributes (raising bias concerns).
- How is user data protected within the model? We know Stripe uses personal data to train its models. But is the foundation model trained in a way that anonymizes inputs and prevents any individual’s data from being extractable? Modern AI can sometimes memorize specific data points (like card numbers) if not properly regularized. Stripe didn’t detail its privacy-preserving techniques. Ensuring that the model doesn’t become a repository of personal info that could be misused or breached is critical. Some companies use techniques like differential privacy in training; did Stripe?
- Will Stripe provide explanations for decisions or a way to appeal them? As discussed, explainability is thin. If a business or an end customer asks “why was this transaction flagged?”, does Stripe have an answer beyond “our model thought it was risky”? Stripe’s documentation for Radar provides reason codes in some cases (like “IP address too far from billing address”), but a complex model might give more opaque reasons. Did Stripe build any interpretable layer or dashboard so that merchants can understand patterns in what’s being blocked? This would build trust and also help merchants tweak their own policies if needed.
- How does the model handle new, emerging fraud tactics? Stripe touted adaptability, but fraudsters will certainly test this model’s limits. If a completely novel scam starts (say, something that wasn’t in the training data at all), how quickly can the foundation model recognize it? Self-supervised learning gives it a broad base, yet some issues might still require human-in-the-loop updates or additional training. Stripe didn’t mention if they have a mechanism for rapid model updates or fine-tuning as new fraud patterns emerge. The concern is whether a foundation model could become too monolithic or slow to adjust in between major training runs, compared to smaller models that can be tweaked more frequently. In an industry where zero-day fraud exploits can cause damage in hours, responsiveness is key.
- What about bias and fairness testing? This was notably absent from Stripe’s public materials. One would hope Stripe’s data scientists ran bias audits – checking if the model’s error rates are higher for transactions involving certain regions, ethnic names, small vs. large merchants, etc. But Stripe didn’t disclose any such analysis or steps taken to mitigate bias. This leaves open the question: Could the model unfairly impact certain groups? For example, could it be tougher on transactions from developing countries, or from low-income ZIP codes, because historically there’s been more fraud there? If so, how will Stripe ensure genuine customers from those places aren’t continually caught in the dragnet? We simply don’t know what fairness safeguards (if any) are in place.
- Is the model “explainable” to regulators and banks? If a bank or regulator audits Stripe, can Stripe provide insights into the model’s decisions? Or is even Stripe’s team treating it as a black box that just empirically works well? The answer might be technical, but important. In high-stakes scenarios (say, a major merchant disputes Stripe’s decline decisions), having some ability to probe the model’s logic could be necessary. Stripe hasn’t said if they’ve incorporated any explainable AI techniques or at least analytic tools to interpret it internally.
- How will this model interact with card network rules and issuer decisions? Sometimes a transaction is blocked by Stripe’s checks before it even goes to the card issuer for approval; other times, Stripe lets it through and the issuer’s system might decline it. If Stripe’s model gets extremely strict, it might decline things that issuers would have approved. That could in theory conflict with network principles of honoring valid transactions. Conversely, if Stripe approves marginal transactions that issuers then decline as fraud, did it just shift the problem downstream? Ideally the model works in harmony with issuers’ models, but Stripe didn’t detail any collaboration with banks or networks on this. (They did mention partnership with Visa on stablecoin payouts, but not on AI decisions.) There’s a gap here: how do Stripe’s risk scores integrate with, or differ from, the risk scores Visa or Mastercard provide to banks? Could a bank say “hey Stripe, you’re letting risky stuff hit us”? Or will they be happy Stripe caught it first? It’s an open question.
- Will merchants get any choice or control? Stripe says the model will be deployed across its suite, implying it’s the new default brain of Stripe’s operations. But merchants vary in their risk tolerance. Some might want ultra-conservative fraud blocking (even if it means a few extra false declines), others might prefer to accept a bit more fraud if it means more sales. Previously, Stripe’s Radar allowed some tuning and custom rules. With a monolithic AI, how much can a merchant adjust? If a business notices the AI is rejecting a certain type of transaction they actually want to allow, can they override it easily? Stripe hasn’t outlined how the foundation model’s outputs can be controlled or configured by end users. Possibly Radar rules can still be layered on top (e.g. “whitelist transactions with X characteristic even if scored high risk”), but that remains to be confirmed. A lack of control could frustrate some sophisticated users.
- Resource usage and costs: Training and running such a model is computationally expensive. Stripe hasn’t said if these AI improvements will increase costs for the company (likely yes, given GPU computing isn’t cheap) and whether any of that might be passed to customers in the future. Right now, these features are part of the service. But one wonders, if Stripe is now doing more heavy lifting per transaction (scoring with a massive model), could that affect pricing or performance? They claim it boosts performance (revenue) enough to justify itself, but it’s worth watching if any new fees or premium offerings emerge around “AI-powered” services.
Many of these questions will only be answered with time and experience. Stripe has essentially asked its users to trust its AI, and in fairness, Stripe has earned a lot of trust over the years by generally delivering solid products. Nonetheless, merchants and analysts will be looking for data. It would not be surprising if in a few months Stripe publishes a follow-up, perhaps a whitepaper or a blog, providing more color on how the model has performed and how they’ve addressed some of these issues. For now, we have to take Stripe’s word on the headline figures and assume they have internally grappled with the subtleties. But as skeptics like to say, “Trust, but verify.” An editorial stance here is that while Stripe’s innovation deserves applause, we should keep asking these tough questions – because AI in finance, if unexamined, can introduce new problems even as it solves old ones.
Innovation vs. Oversight: The Road Ahead
Stripe’s foray into foundation models marks a significant moment in fintech – it showcases the immense potential of data-driven AI to improve financial services, while also throwing into relief the responsibilities that come with such power. The editorial view on this development is necessarily mixed: there’s admiration for the technological feat and its promised benefits, and caution about the broader implications for the industry and society.
On one hand, Stripe is pushing the envelope in exactly the way a tech-forward company should. It identified a competitive edge – its colossal dataset – and leveraged modern AI techniques to capitalize on it for the benefit of its users. If fraud gets noticeably harder and legitimate commerce flows more smoothly as a result, Stripe will have delivered value not just to its customers but to consumers and the economy at large. Fewer scams and fewer false declines mean a more efficient market, less waste, and greater trust in online transactions. That is a laudable outcome. It also exemplifies how fintech can innovate faster than traditional banking, by borrowing the playbook of Silicon Valley (build a general AI model, akin to how tech giants do) and applying it to a finance domain problem. This competitive pressure is healthy – it forces everyone in the payments ecosystem to step up. We can expect Visa, banks, and rival fintechs to innovate in response, potentially leading to an arms race of good AI fighting bad AI, with genuine users hopefully winning out.
On the other hand, the deployment of an opaque, powerful AI across a significant chunk of the world’s transactions raises a flag for strong oversight and transparency. When a single platform like Stripe handles ~1.3% of global GDP in transactions and now applies a centralized AI model to much of it, we are witnessing a concentration of influence. Decisions made by Stripe’s algorithms could subtly shape commerce – determining which transactions fail or which merchants face more fraud risk. It puts a lot of trust in Stripe’s internal governance of AI: how they test it, monitor it, and correct it. External regulators will need to keep pace. This is a microcosm of the larger societal issue with AI: innovation often outruns regulation. Stripe’s model will likely be in the wild, learning and affecting outcomes, long before any specific rules (in the US at least) spell out what it can or cannot do. That means Stripe must be its own referee to a large extent, upholding ethical standards and not just profit motives.
Encouragingly, the business incentives do align somewhat with doing the right thing – Stripe gains nothing by accidentally blocking good transactions or angering users with biased outcomes; its success hinges on being accurate and fair. But some friction can occur between maximizing fraud catch and being fair to edge cases. Here, transparency can help: if Stripe engages openly with merchants, regulators, and maybe even publishes performance metrics (like false positive rates, or bias audits), it would set a positive precedent. In an editorial sense, one could argue that Stripe, given its scale, should lead in establishing norms for ethical AI in payments. This could include inviting third-party audits of its model, sharing learnings with the industry on combating bias, or providing channels for consumer feedback on AI-driven decisions. Being proactive could stave off heavy-handed regulation later and build even greater trust.
Another forward-looking implication: Will foundation models trained on proprietary data become the norm in fintech? If Stripe’s model proves a competitive advantage, others will follow suit – perhaps not immediately, but over years. We might see, for instance, large banks federating their data to train joint AI models, or card networks enhancing their global models to not be outdone. There’s even a scenario where regulators or industry groups might push for collaboration: could an industry-wide foundation model (managed by a consortium) serve as a utility for fraud detection? Or would each player insist on their own to differentiate? If each major payment provider runs its own giant AI, they might all catch most fraud but also sometimes conflict in decisions. For developers and merchants, it could become confusing if different platforms have different “AI personalities” – one might decline a transaction that another approves. Over time, convergence or at least interoperability standards might be needed (for example, standard codes for why an AI blocked something, so that can be communicated across the chain).
One cannot ignore the regulatory transatlantic gap: as we noted, Europe is more prescriptive. If the EU’s AI Act comes into force in the next couple of years with stringent requirements, Stripe’s model could become a case study for compliance. Perhaps Stripe will have to significantly document and adjust it to be allowed in the EU market. That compliance burden in turn could shape what the model looks like globally (companies sometimes adopt the highest standard globally to simplify operations). It might also create a competitive opening – if some smaller competitor can’t afford that compliance, Stripe’s leadership solidifies; or conversely, if Stripe struggles with EU requirements, maybe a European-born solution finds favor with those who want a more transparent tool. The UK, EU, and US may diverge in how far they let such AI go without oversight, and Stripe will be navigating all three environments. Watching how Stripe adapts to each will be telling for the future of AI in fintech.
For fintech startups broadly, Stripe’s move reinforces a trend: the big get bigger (in data) and smarter (in AI), so newcomers must find creative angles. Perhaps the next wave of fintech innovation will involve leveraging AI but in more specialized ways, or focusing on aspects like user experience around AI decisions – areas where a giant platform might be too generic. There’s also an argument that open banking and data portability (which regulators in Europe champion) could provide counterweight: if merchants or consumers could easily share data with multiple providers, maybe no single platform has all the data. But in reality, Stripe’s scale gives it a moat that’s hard to breach.
In closing, Stripe’s new AI foundation model is both an impressive achievement and a reminder of the duality of technology – the same tool that can reduce fraud by double digits can also stoke fears of unchecked algorithms. The challenge and opportunity now is to integrate such AI into the financial system in a way that enhances trust. Stripe likely understands that its long-term success with this AI will depend not just on raw performance, but on the comfort level of users, merchants, and regulators with how it operates. That means being open about results, responsive to concerns, and vigilant that the model serves all stakeholders fairly.
As Stripe leads the charge, it’s setting precedents. If it succeeds and does so responsibly, it will underscore how thoughtful innovation can make commerce better for everyone. If it stumbles – say, through a public mishap or regulatory penalty – it will serve as a cautionary tale that even well-intentioned AI can go awry without proper checks. The editorial perspective finds optimism in Stripe’s track record and the clear benefits on paper, but also urges continuous scrutiny. In the end, it’s a grand experiment: Can an AI trained on the world’s payments make the internet economy meaningfully safer and smoother, without sacrificing privacy or fairness? The answer, unfolding in real time, will likely influence fintech strategies and AI policies for years to come. Stripe has made its move; now the world will be watching – and learning – as the outcomes emerge.